How to triple your return on ad spend using predictive modelling
And how it really works
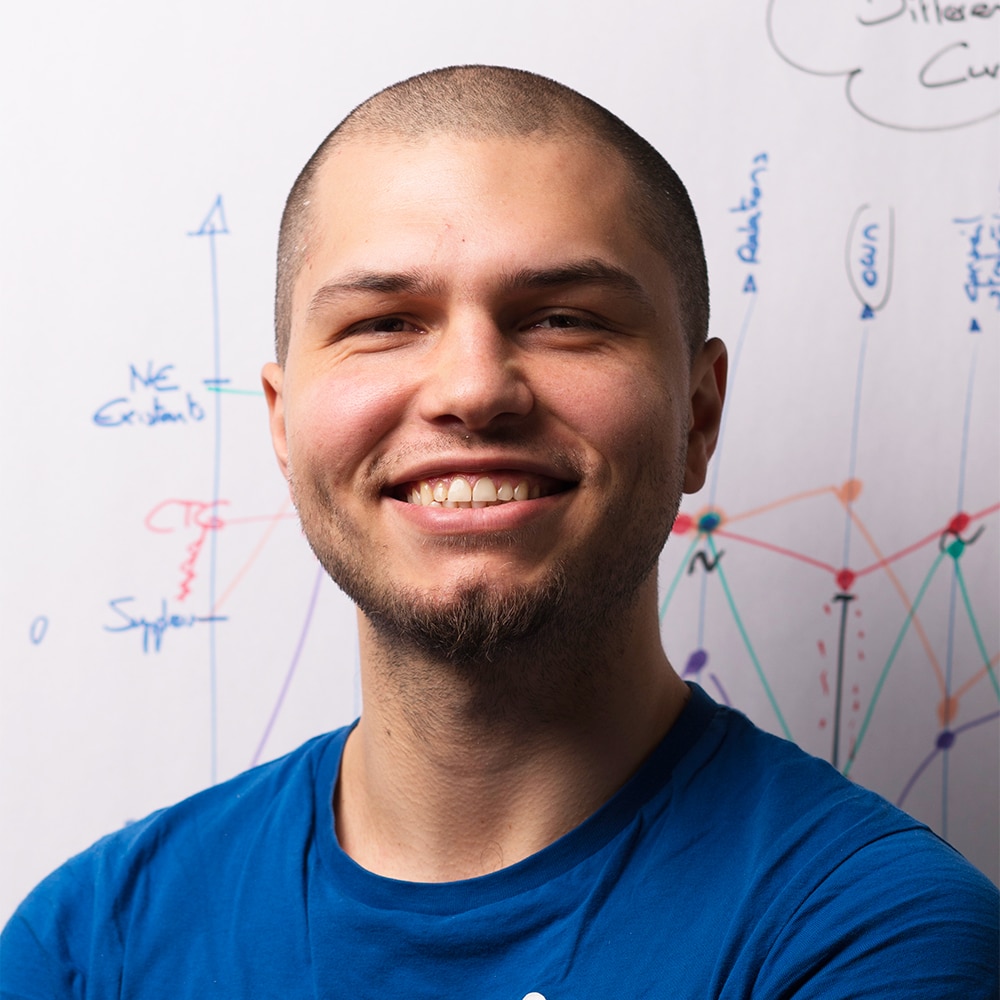
By ARBEN KQIKU
Predictive modelling helps overcome the challenges of scale when allocating and optimising ad spend across ever-expanding ad portfolios. Here’s how it works.
Allocating and redistributing advertising budgets weekly can be a difficult and time-consuming task, especially when dealing with dozens or even hundreds of global ad campaigns or multiple channels. That being said, failing to optimise ad spend and allocate budgets effectively can lead to a great deal of wasted money.
The key goal is to distribute budgets in a way that generates the most possible value for the business. Therefore, we developed an algorithm to enable seamless budget distribution and optimization. Working with a client who was running 170 Google Ads worldwide, this approach allowed us to increase the average return on advertising spend (ROAS) from 2.8 to 8.4 in less than two months.
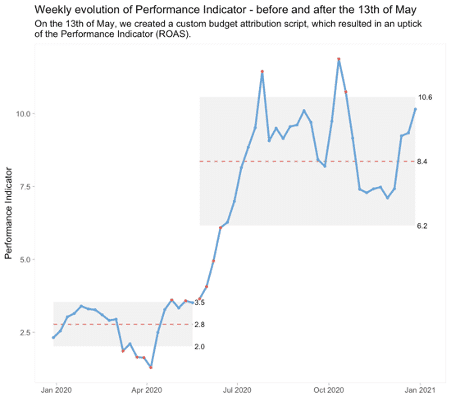
Here’s how the algorithm works:
We used predictive modelling based on past data to optimise budget allocation. Here are the factors that we used in our model to achieve a three-fold increase in ROAS:
- Data normalisation: Different campaigns typically have different durations and starting dates, so we needed to bring all these together on the same scale before we could compare them.
- Conversion rate: This helps us estimate a campaign’s ability to convert users into customers and, consequently, prioritise those that generate the most customers.
- Conversion value: This helps us estimate the amount of revenue generated by each campaign, thereby allowing us to prioritise those that generate the most revenue.
- ROAS: The return on advertising spend allows us to estimate the profitability of each ad campaign, again allowing us to prioritise the most profitable ones.
- Adjustment factor: Expectations must vary depending on the stage of the ad campaign, whether it is the top of the funnel (TOFU), the middle of the funnel (MOFU), or the bottom of the funnel (BOFU). Therefore, if a campaign performs better than average at a certain stage of the funnel, we can reallocate budgets accordingly.
- Impression share: This allows us to estimate the growth potential of a campaign. Some campaigns have already reached their peaks in terms of impressions, while others still have a huge growth potential. If we estimate that a campaign has a large potential growth margin, then a higher portion of the budget will be allocated to it.
- Minimal budget: Finally, we calculate the minimum budget for each campaign category (TOFU, MOFU, or BOFU). This allows us to guarantee a minimal level of brand visibility, although the main goal is performance.
Final words
Every business has limited resources. There are multiple active advertising channels, and within each of these there are myriad activities or campaigns. By using historical data, we can build predictive models to make an informed estimate as to which campaigns and channels can realistically be expected to perform the best. These models allow us to allocate advertising budgets effectively, thus increasing performance and profitability while reducing risk.
While such algorithms offer enormous value by saving time and money, it is still important to be aware of their limitations. For example, predictive models are based on past performance, which means that any changes made to the campaign afterwards will not be considered. This is why it is important to consider human modifications as well. Furthermore, it is important to measure everything properly. After all, you can’t improve what you’re not measuring.
While we used to spend three hours per week just managing ad budgets for this particular client, we can now manage everything better and faster in the background. This frees up time to focus on strategy, thereby maximising the effectiveness of the ad campaigns across the board.
We adapt our approach to meet the unique needs of every client. After all, predictive models must be tailored to your data, your business, and your specific goals. If you are having a hard time allocating resources efficiently, get in touch today so we can build a custom solution that works for you.